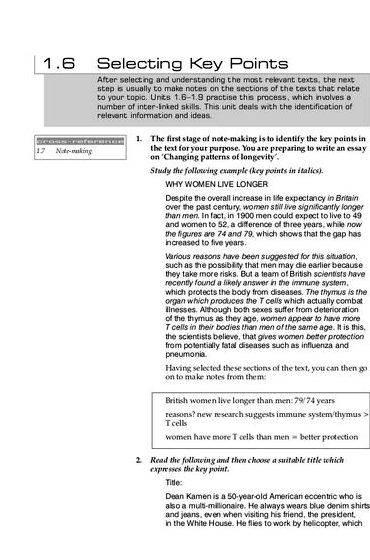
Jan 12, · [45] M. W. Dixon, "Application of neural networks to solve the routing problem in communication networks," Ph.D. dissertation, Murdoch Univ., Murdoch, WA, Australia, Published Thesis [46] [46] M. Lehmann, Data Access in Workflow Management Systems. Berlin: Aka, Thesis from a Full Text Database [47] Apr 13, · (a) Principal component analysis as an exploratory tool for data analysis. The standard context for PCA as an exploratory data analysis tool involves a dataset with observations on p numerical variables, for each of n entities or individuals. These data values define p n-dimensional vectors x 1,,x p or, equivalently, an n×p data matrix X, whose jth column is the vector x j of observations Educational technology (commonly abbreviated as EduTech, or EdTech) is the combined use of computer hardware, software, and educational theory and practice to facilitate learning. When referred to with its abbreviation, EdTech, it is often referring to the industry of
Principal component analysis: a review and recent developments
Try out PMC Labs and tell us what you think. Learn More. The fossil teeth data are available from I. Large datasets are increasingly common and are often difficult to interpret. Principal component analysis PCA is a technique for reducing the dimensionality of such datasets, increasing interpretability but at the same time minimizing information loss. It does so by creating new uncorrelated variables that successively maximize variance.
It is adaptive in another sense too, since variants of the technique have been developed that are tailored to various different data types and structures. This article will begin by introducing the basic ideas of PCA, discussing what it can and cannot do, online dissertation help berlin.
It will then describe some variants of PCA and their application. Large datasets are increasingly widespread in many disciplines. In order to interpret such datasets, methods are required to drastically reduce their dimensionality in an interpretable way, such that most of the information in the data is preserved. Many techniques have been developed for this purpose, but principal component analysis PCA is one of the oldest and most widely used.
statistical information as possible. Although it is used, and has sometimes been reinvented, in many different disciplines it is, at heart, a statistical technique and hence much of its development has been by statisticians. The earliest literature on PCA dates from Pearson [ 1 ] and Hotelling [ 2 ], but it was not until electronic computers became widely available decades later that it was computationally feasible to use it on datasets that were not trivially small.
Since then its use has burgeoned and a large number of variants have been developed in many different online dissertation help berlin. Substantial books have been written on the subject [ 34 ] and there are even whole books on variants of PCA for special types of online dissertation help berlin [ 56 ]. In § 2the formal definition of PCA will be given, in a standard context, together with a derivation showing that it can be obtained as the solution to an eigenproblem or, alternatively, from the singular value decomposition SVD of the centred data matrix, online dissertation help berlin.
PCA can be based on either the covariance matrix or the correlation matrix. The choice between these analyses will be discussed. In either case, the new variables the PCs depend on the dataset, rather than being pre-defined basis functions, and so are adaptive in the broad sense. The main uses of PCA are descriptive, rather than inferential; an example will illustrate this. Although for inferential purposes a multivariate normal Gaussian distribution of the dataset is usually assumed, PCA as a descriptive tool needs no distributional assumptions and, as such, is very much an adaptive exploratory method which can be used on numerical data of various types.
Indeed, many adaptations of the basic methodology for different data types and structures have been developed, two of which will be described in § 3 a,d. Some techniques give simplified versions of PCs, in order to aid interpretation. Two of these are briefly described in § 3 b, which also includes an example of PCA, together with a simplified version, in atmospheric science, illustrating the adaptive potential of PCA in a specific context.
Section 3 c discusses one of the extensions of PCA that has been most active in recent years, namely robust PCA RPCA.
The explosion in very large datasets in areas such as online dissertation help berlin analysis or the analysis of Web data has brought about important methodological advances in data analysis which often find their roots in PCA. Each of § 3 a—d gives references to recent online dissertation help berlin. Some concluding remarks, emphasizing the breadth of application of PCA and its numerous adaptations, are made in § 4.
The standard context for PCA as an exploratory data analysis tool involves a dataset with observations on p numerical variables, for each of n entities or individuals. These data values define p n -dimensional vectors x 1 ,…, x p or, equivalently, an n × p data matrix Xwhose j th column is the vector x j of observations on the j th variable.
We seek a linear combination of the columns of matrix X with maximum variance. Such linear combinations are given bywhere a is a vector of constants a 1a 2 ,…, a p. For this problem to have a well-defined solution, an additional restriction must online dissertation help berlin imposed and the most common restriction involves working with unit-norm vectors, i.
Differentiating with respect to the vector aand equating to the null vector, produces the equation. Thus, a must be a unit-norm eigenvector, and λ the corresponding eigenvalue, of the covariance matrix S, online dissertation help berlin. Equation 2.
A Lagrange multipliers approach, with the added restrictions of orthogonality of different coefficient vectors, online dissertation help berlin, can also be used to show that the full set of eigenvectors of S are the solutions to the problem of obtaining up to p new linear combinationswhich successively maximize variance, subject to uncorrelatedness with previous linear combinations [ 4 ].
In standard PCA terminology, the elements of the eigenvectors a k are commonly called the PC loadingswhereas the elements of the linear combinations X a k are called the PC scoresas they are the values that each individual would score on a given PC.
This convention does not change the solution other than centringsince the covariance matrix of a set of centred or uncentred variables is the same, but it has the advantage of providing a direct connection to an alternative, online dissertation help berlin geometric approach to PCA.
Any arbitrary matrix Y of dimension n × p and rank r necessarily, can be written e, online dissertation help berlin. We assume that the diagonal elements of L are in decreasing order, and this uniquely defines the order of the columns of U and A except for the case of equal singular values [ 4 ].
Equivalently, and given 2. where L 2 is the diagonal matrix with the squared singular values i. The properties of an SVD imply interesting geometric interpretations of a PCA. where L q is online dissertation help berlin q × q diagonal matrix with the first largest q diagonal elements of L and U qA q are the n × q and p × q matrices obtained by retaining the q corresponding columns in U and A, online dissertation help berlin.
The system of q axes in this representation is given by the first q PCs and defines a principal subspace. Hence, PCA is at heart a dimensionality-reduction method, whereby a set of p original variables can be replaced by an optimal set of q derived variables, the PCs. The quality of any q -dimensional approximation can be measured by the variability associated with the set of retained PCs, online dissertation help berlin.
In fact, the sum of variances of the p original variables is the trace sum of diagonal elements of the covariance matrix S. Using simple matrix theory results it is straightforward to show that this value is also the sum of the variances of all p PCs. Hence, the standard measure of quality of a given PC is the proportion of total variance that it accounts for. where tr S denotes the trace of S.
The incremental nature of PCs also means that we can speak of a proportion of total variance explained by a set of PCs usually, but not necessarily, the first q PCswhich is often expressed as a percentage of total variance accounted for:.
Even in such situations, the percentage of total variance accounted for is a fundamental tool to assess the quality of these low-dimensional graphical representations of the dataset, online dissertation help berlin. The emphasis in PCA is almost always on the first few PCs, online dissertation help berlin, but there are circumstances in which the last few may be of interest, such as in outlier detection [ 4 ] or some applications of image analysis see § 3 c.
PCs can also be introduced as the optimal solutions to numerous other problems. Optimality criteria for PCA are discussed in detail in numerous sources see [ 489 ], among others. McCabe [ 10 ] uses some of these criteria to select optimal subsets of the original variables, which he calls principal variables. This is a different, computationally more complex, online dissertation help berlin, problem [ 11 ].
PCA has been applied and found useful in very many disciplines. The two examples explored here and in § 3 b are very different in nature. The first examines a dataset consisting of nine measurements on 88 fossil teeth from the early mammalian insectivore Kuehneotherium, while the second, in § 3 b, is online dissertation help berlin atmospheric science.
Kuehneotherium is one of the earliest mammals and remains have been found during quarrying of limestone in South Wales, online dissertation help berlin, UK [ 12 ]. The bones and teeth were washed into fissures in the rock, about million years ago, and all the lower molar teeth used in this analysis are from a single online dissertation help berlin. However, it looked possible that there were teeth from more than one species of Kuehneotherium in the sample.
Of the nine variables, three measure aspects of the length of a tooth, while the other six are measurements related online dissertation help berlin height and width. A PCA was performed using the prcomp online dissertation help berlin of the R statistical software [ 13 ]. The first two PCs account for In figure 1large teeth are on the left and small teeth on the right.
Fossils near the top of figure 1 have smaller lengths, relative to their heights and widths, than those towards the bottom. The relatively online dissertation help berlin cluster online dissertation help berlin points in the bottom half of figure 1 is thought to correspond to a species of Kuehneotherium, while the broader group at the top cannot be assigned to Kuehneotherium, but to some related, but as yet unidentified, animal.
The two-dimensional principal subspace for the fossil teeth data. The coordinates in either or both PCs may switch online dissertation help berlin when different software is used.
So far, PCs have been presented as linear combinations of the centred original variables. However, the properties of PCA have some undesirable features when these variables have different units of measurement.
While there is nothing inherently wrong, from a strictly mathematical point of view, with linear combinations of variables with different units of measurement their use is widespread in, for instance, linear regressiononline dissertation help berlin, the fact that PCA online dissertation help berlin defined by a criterion variance that depends on units of measurement implies that PCs based on the covariance matrix S will change if the units of measurement on one or more of the variables change unless all p variables undergo a common change of scale, in which case the new covariance matrix is merely a scalar multiple of the old one, hence with the same eigenvectors and the same proportion of total variance explained by each PC.
To overcome this undesirable feature, it is common practice to begin by standardizing the variables. Each data value x ij is both centred and divided by the standard deviation s j of the n observations of variable j. Thus, the initial data matrix X is replaced with the standardized data matrix Zwhose j th column is vector z j with the n standardized observations of variable j 2. Standardization online dissertation help berlin useful because most changes of scale are linear transformations of the data, online dissertation help berlin, which share the same set of standardized data values.
Since the covariance matrix of a standardized dataset is merely the correlation matrix R of the original dataset, a PCA on the standardized data is also known as a correlation matrix PCA. The eigenvectors a k of the correlation matrix R define the uncorrelated maximum-variance linear combinations of the standardized variables z 1 ,…, z p. Such correlation matrix PCs are not the same as, nor are they directly related to, the covariance matrix PCs defined previously.
Also, the percentage variance accounted for by each PC will differ and, quite frequently, more correlation matrix PCs than covariance matrix PCs are needed to account for the same percentage of total variance.
The trace of a correlation matrix R is merely the number p of variables used in the analysis, hence the proportion of total variance accounted for by any correlation matrix PC is just the variance of that PC divided by p. The SVD approach is also valid in this context. Correlation matrix PCs are invariant to linear changes in units of measurement and are therefore the appropriate choice for datasets where different changes of scale are conceivable for each variable, online dissertation help berlin.
In a correlation matrix PCA, the coefficient of correlation between the j th variable and the k th PC is given by see [ 4 ]. In the fossil teeth data of § 2 b, all nine measurements are in the same units, online dissertation help berlin a covariance matrix PCA makes sense. A correlation matrix PCA produces similar results, since the variances of the original variable do not differ very much.
The first two correlation matrix PCs account for For other datasets, differences can be more substantial. One of the most informative graphical representations of a multivariate dataset is via a biplot [ 14 ], which is fundamentally connected to the SVD of a relevant data matrix, and therefore to PCA, online dissertation help berlin. The n rows g i of matrix G define graphical markers for each individual, which are usually represented by points.
The p rows h j of matrix H define markers for each variable and are usually represented by vectors. The practical implication of this result is that orthogonally projecting the point representing individual i onto the vector representing variable j recovers the centred value. Figure 2 gives the biplot for the correlation matrix PCA of the fossil teeth data of § 2 b.
Degrees For Sale: Inside The Essay Writing Industry: Students On The Edge
, time: 5:34All Examples - IEEE - Referencing Guide - Help and Support at Murdoch University
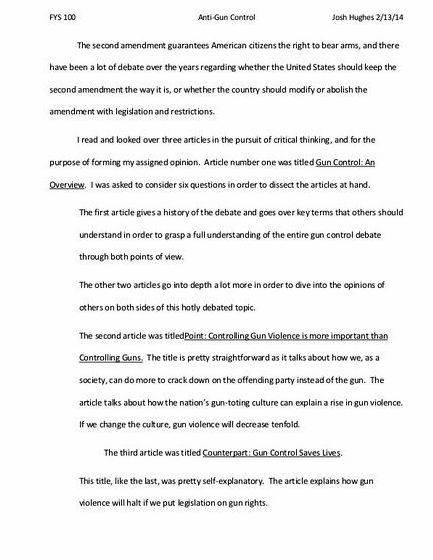
Educational technology (commonly abbreviated as EduTech, or EdTech) is the combined use of computer hardware, software, and educational theory and practice to facilitate learning. When referred to with its abbreviation, EdTech, it is often referring to the industry of Jan 12, · [45] M. W. Dixon, "Application of neural networks to solve the routing problem in communication networks," Ph.D. dissertation, Murdoch Univ., Murdoch, WA, Australia, Published Thesis [46] [46] M. Lehmann, Data Access in Workflow Management Systems. Berlin: Aka, Thesis from a Full Text Database [47] Apr 13, · (a) Principal component analysis as an exploratory tool for data analysis. The standard context for PCA as an exploratory data analysis tool involves a dataset with observations on p numerical variables, for each of n entities or individuals. These data values define p n-dimensional vectors x 1,,x p or, equivalently, an n×p data matrix X, whose jth column is the vector x j of observations
No comments:
Post a Comment